Trading, whether under a buttonwood tree on Wall Street in the 18th century or in a New Jersey data center in the 21st, has always been about making quick calculations. But these days, getting an edge requires the most talented mathematical brains, which are in high demand from Silicon Valley to Singapore.
Nicolai Meinshausen, 43, a statistics professor at ETH Zurich who has racked up a series of awards (pdf), is among the talent that trading firms are looking to woo. Citadel Securities, one of the biggest market makers in the world, has hired the machine-learning expert as a senior fellow and head of principal research. Meinshausen’s research focuses on the intersection of machine learning and causality. He analyzes vast troves of market data to make predictions about when and where the company should be buying and selling financial assets.
A handful of statisticians who are at the top of the field can crack challenges that seem otherwise out of reach. The opportunity to take on the thorniest problems is what lures these number crunchers, who have their pick of jobs in a wide range of sectors. Meinshausen said the financial industry offers more interesting challenges for a mathematician to tackle than others. “Quantitative trading firms like Citadel Securities have access to unparalleled amounts of data,” said Meinshausen, who was formerly a post-doctoral fellow at Berkeley and a professor of statistics at Oxford. “This presents immense opportunities for the application of machine learning and advanced statistical analysis.”
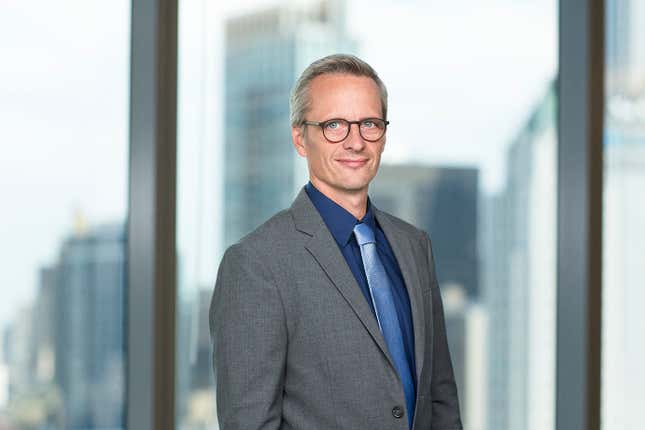
Quant strategies have become increasingly popular, and Citadel is far from the only trading company courting top mathematicians. XTX, whose name refers to the linear regression formula, said this summer that it was running an online contest with a $30,000 cash prize to find promising data scientists. (The London-based trading firm’s office is a temple to the gods of math.) In New York, Hudson River Trading’s university recruiting schedule shows it making the rounds to more than a dozen of the world’s top-tier engineering schools.
There’s a finite pool of top mathematicians and statisticians, and trading firms are competing for their services with deep-pocketed tech giants, Wall Street banks, and startups that can promise (potentially) lucrative stock options. “College graduates may not know these trading-firm brands as well so they may have to work harder,” said Kevin McPartland, head of market structure and technology research at Greenwich Associates. Luring a star can help a company attract others who are eager to work with that person.
From options to Spotify
Citadel Securities, which is separate from the $30 billion hedge fund Citadel also started by Ken Griffin, started out in 2002. In its early days it was an options market maker, posting electronic bids and offers so that investors have a ready market where they can transact. Over the years it branched into stocks, interest-rate swaps, Treasuries, and became a direct market maker—tasked with making sure trading stays orderly—on the New York Stock Exchange, where it handled Spotify and Slack’s highly publicized direct listings. It says it takes part in 20% of US stock trading each day.
A statistics expert now runs the trading firm. Beijing-born Peng Zhao got his PhD at the University of California in Berkeley, where he overlapped for a time with Meinshausen, and he became CEO two years ago.
The financial industry obviously pays better than academia. The trading business also offers something else that universities can’t: Researchers are able to quickly see their results. Don Wilson, the founder of another global trading firm named DRW, was a student at University of Chicago when he realized that trading made academia seem comparatively frustrating.
“You can build a mathematical model and then try it out, and see if it works,” he said in a speech. “And that seemed so much more interesting than writing an academic paper, debating whether you’re right or wrong. Two years later you may never know.”
The immediacy of testing algorithms in live financial markets was also appealing to Meinshausen, who pointed out that he will still work part-time at the university. “I wanted the opportunity to not only explore and understand ideas but to also apply solutions and quickly see the results come to fruition,” he said. “At the same time, I still had the ability to conduct long-term analysis, which is similar to academia.”
Upping the ante
Trading, meanwhile, is more competitive than ever. As electronic buying and selling becomes more efficient, there’s less money left over for market makers. Financial market volatility has also been more muted in recent years, making it more difficult for traders to eke out a profit.
“All you see is consolidation and people are leaving the market,” said Larry Tabb, founder and research chairman at Tabb Group. “The expense of getting in is very high. The ante has gone up, and the pot of winnings has gone down.”
Keeping up requires heavy investment. Only a handful of firms can still compete in “low latency” strategies, or truly high frequency trading, where the majority of the profit goes to a few firms that can afford the technology needed to act on millisecond price changes. Citadel, which is among the biggest and most diversified traders, is a private company and doesn’t disclose its profits.
Quant trading is no guarantee of blockbuster profits, but it’s growing in popularity. John Lin, the founder of Singapore-based Grasshopper, said he was skeptical that machine-learning strategies would work when markets inevitably encounter paradigms that didn’t fit the databases they were trained on. “I’ve learned there is an art to it,” Lin, an engineering grad from Cornell who started out as a clerk at the Chicago Mercantile Exchange, said in an email.
“Grasshopper itself has shifted radically towards heavy data-driven strategies,” he said. “They work, especially in well tested pockets that generate clean data, and can be quite consistently profitable. But you need scale to make real money.”
In the meantime, trading companies will continue to hoard brain power. Fortunately for them, they have several attractions to lure the top number crunchers, like the chance to work with colleagues who are top statisticians, as well as the opportunity to crack difficult problems. “The ability to work with large and complex datasets is very exciting for someone with my background,” Meinshausen said.